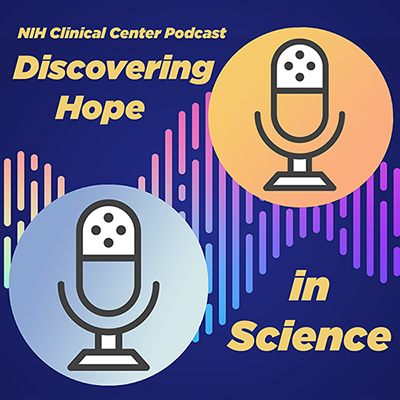
In this episode, we hear from Dr. Ronald Summers, a tenured Senior Investigator and Staff Radiologist in the Clinical Center's Radiology and Imaging Sciences Department, on how scientists succeeded in using Artificial Intelligence technology to assist in lymph node detection. This detection can be critical in assessing lymphadenopathy (swelling of lymph nodes) and may lead to a better control of infection and cancer spread (metastases).
Listen and Subscribe to Discovering Hope in Science
On this page:
Featured Speaker: Dr. Ronald M. Summers | Show Notes | Episode Transcript
Featured Speaker: Dr. Ronald M. Summers
Dr. Ronald M. Summers is a pioneer in the use of artificial intelligence in radiology. His lab has made seminal contributions to the advancement of cancer diagnosis using radiology.
Dr. Summers received a BA degree in physics and MD and PhD degrees in Medicine/Anatomy and Cell Biology from the University of Pennsylvania. He completed a medical internship at the Presbyterian-University of Pennsylvania Hospital, Philadelphia, PA, a radiology residency at the University of Michigan, Ann Arbor, MI, and an MRI fellowship at Duke University, Durham, NC. In 1994, he joined the Radiology and Imaging Sciences Department at the NIH Clinical Center where he is now a tenured senior investigator and staff radiologist. Read his full bio.
Show Notes
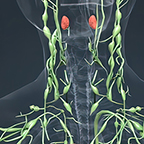
- Dr. Ronald M. Summers, MD, PhD
- Duke University
- Presbyterian-University of Pennsylvania Hospital
- Radiology and Imaging Sciences Department
- Universal Lymph Node Detection in T2 MRI Using Neural Networks
- University of Michigan
- University of Pennsylvania
Episode Transcript
[Background Music]
DR RONALD M. SUMMERS: If we're trying to find lymph nodes anywhere in the body, the neural network needs to learn what normal anatomy everywhere in the body looks like. This ups the complexity of the challenge because the body, the human anatomy, varies very much from one part of the body to another. The training is similar: data is fit into the neural network during the training phase. The network then adjusts the weights.
NARRATOR: From the National Institutes of Health Clinical Center, this is Discovering Hope in Science.
[Background Music]
DAN SILBER: My name is Dan Silber from the Office of Communications and Media Relations, and I'll be your host. Today we'll be talking about the article "Universal Lymph Node Detection in T2 MRI Using Neural Networks."
Dr. [Ronald M.] Summers is a tenured senior investigator and staff radiologist in the Radiology and Imaging Sciences Department at the NIH Clinical Center in Bethesda, Maryland. His research interests include thoracic and abdominal imaging, large radiology Image databases, and artificial intelligence. Dr. Summers - thank you so much for being with us today.
SUMMERS: It's a great pleasure to be here with you.
SILBER: Today we're talking about the article 'Universal lymph node detection in T2 MRI using neural networks'. For those that are unfamiliar, what's a neural network?
SUMMERS: So a neural network is a set of mathematical formula arranged in layers that take in data and output predictions. So for example in this case, the data that's being taken in are MRI scans and the predictions that come out the other end are the locations of abnormalities - like lymph nodes.
SILBER: What's the advantage of using neural networks over an actual human being?
SUMMERS: That's a good question. Trained humans are great at doing this task - but trained humans have to go through years of education. Whereas once a computer program is trained, it can be copied and reproduced and can provide services at lower costs than otherwise might be required with a human.
SILBER: So how do you train those neural networks?
SUMMERS: In our field, we'll take images with labels showing the locations of the lymph nodes, and we will then feed a large number of them into the computer. During the training process, the neural network learns where lymph nodes are likely to be and what they typically look like. And then, once the training is done, we will then evaluate how well the neural network works with data that the neural network hasn't seen before.
SILBER: That's very cool. Is that time consuming?
SUMMERS: The training can take sometimes days or even weeks, but once the network is trained, the network can make the predictions very quickly, sometimes in less than a second.
SILBER: How are neural networks in healthcare different than art or in writing production, which also use neural networks?
SUMMERS: There are many similarities. Just like in the examples that you mentioned, the training is similar: data is fed into the neural network during the training phase. The network then adjusts the weights inside the network and then predictions are made, which are then tested in a fresh set of data - and we do the same thing with healthcare data. We train using images or other medical data in which the predictions are known, and then evaluate whether the network does well with images that were not provided during the training.
SILBER: In this case how does lymph node universal detection differ from finding lymph nodes in some specific part of the body?
SUMMERS: So in universal detection, we're trying to find every lymph node anywhere in the body whereas in earlier works we were focusing on particular areas in the body. This ups the complexity of the challenge because the body - the human anatomy - varies very much from one part of the body to another. And when we're trying to find lymph nodes anywhere in the body, the neural network needs to learn what normal anatomy everywhere in the body looks like.
SILBER: How does this work? How will that trickle down and affect the population as a whole?
SUMMERS: The hope is that once these systems are trained they can be used as part of daily patient care, in order to provide more accurate detections of lymph nodes. Another important application, particularly in patients with cancer, is that instead of measuring or evaluating just a couple of lymph nodes. The computer can analyze every lymph node in the entire body, and that permits things like measuring the total tumor burden: Something that today is very difficult to do, but I believe in the future will be a useful thing.
SILBER: Thank you so much for joining us today and discussing your research with us! Thanks for listening to Discovering Hope in Science. View more episodes.